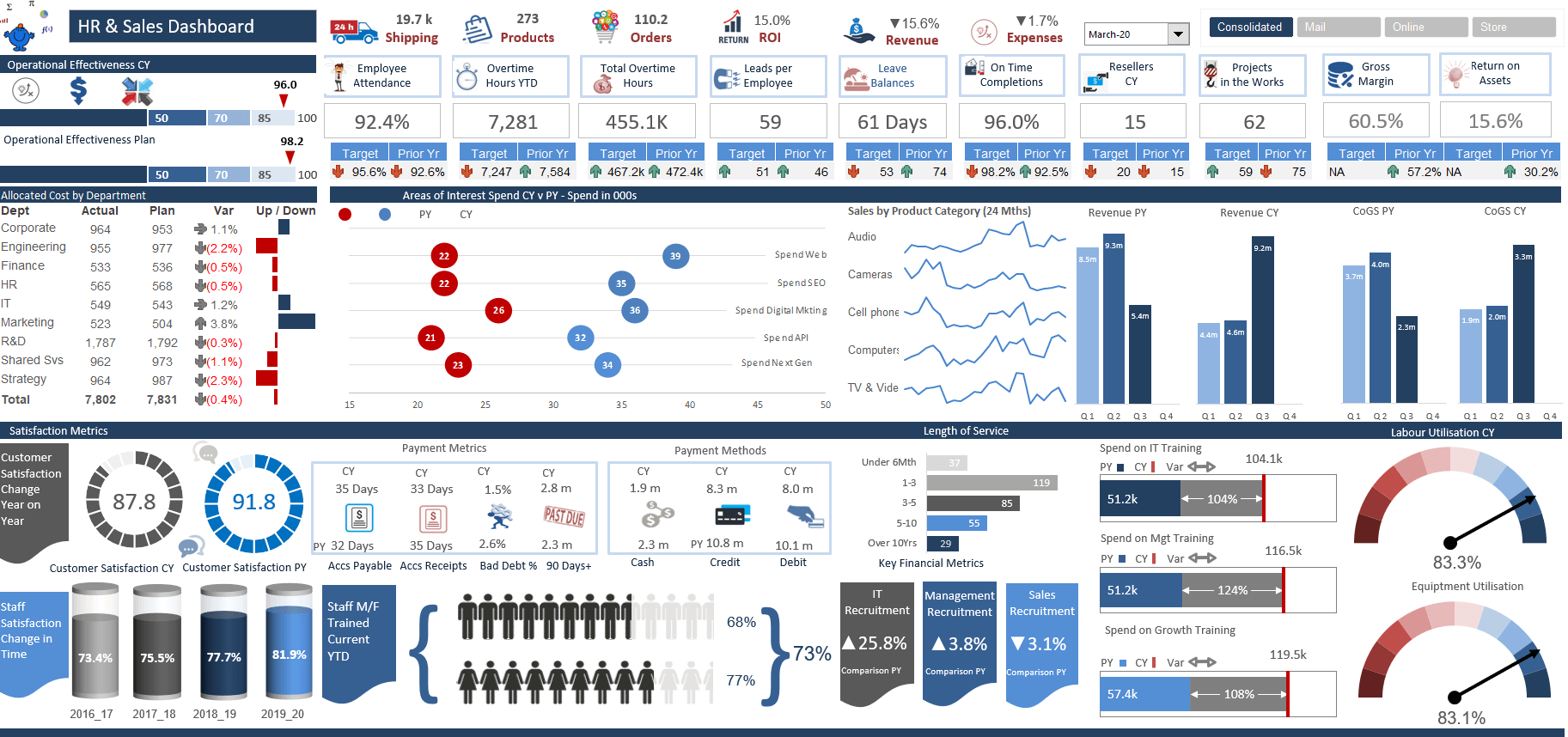
Data analytics: Everyone’s talking about it, but what exactly is it? Perhaps you’ve heard that data analytics is the next big thing for business and want to finally understand what all the hype is about. Maybe you’re thinking about becoming a data analyst and want to know what a career in the field really involves. Either way, you’ve come to the right place! In this guide, you’ll find a complete and comprehensive introduction to data analytics—starting with a simple, easy-to-understand definition and working up to some of the most important tools and techniques. We’ll also touch upon how you can start a career as a data analyst, and explore what the future holds in terms of market growth. By the end of this post, you’ll have a clear idea of what data analytics is, how it differs from data science, and what it takes to break into the field. Want to skip ahead to a specific section? Just use the clickable menu below.
1. What is data analytics?
Most companies are collecting loads of data all the time—but, in its raw form, this data doesn’t really mean anything. This is where data analytics comes in. Data analytics is the process of analyzing raw data in order to draw out meaningful, actionable insights. These insights are then used to inform and drive smart business decisions. So, a data analyst will extract raw data, organize it, and then analyze it, transforming it from incomprehensible numbers into coherent, intelligible information. Having interpreted the data, the data analyst will then pass on their findings in the form of suggestions or recommendations about what the company’s next steps should be. You can think of data analytics as a form of business intelligence, used to solve specific problems and challenges within an organization. It’s all about finding patterns in a dataset which can tell you something useful and relevant about a particular area of the business—how certain customer groups behave, for example, or how employees engage with a particular tool. Data analytics helps you to make sense of the past and to predict future trends and behaviors; rather than basing your decisions and strategies on guesswork, you’re making informed choices based on what the data is telling you. Armed with the insights drawn from the data, businesses and organizations are able to develop a much deeper understanding of their audience, their industry, and their company as a whole—and, as a result, are much better equipped to make decisions and plan ahead.
2. What’s the difference between data analytics and data science?
You’ll find that the terms “data science” and “data analytics” tend to be used interchangeably. However, they are two different fields and denote two distinct career paths. What’s more, they each have a very different impact on the business or organization. One key difference between data scientists and data analysts lies in what they do with the data and the outcomes they achieve. A data analyst will seek to answer specific questions or address particular challenges that have already been identified and are known to the business. To do this, they examine large datasets with the goal of identifying trends and patterns. They then “visualize” their findings in the form of charts, graphs, and dashboards. These visualizations are shared with key stakeholders and used to make informed, data-driven strategic decisions. A data scientist, on the other hand, considers what questions the business should or could be asking. They design new processes for data modeling, write algorithms, devise predictive models, and run custom analyses. For example: They might build a machine to leverage a dataset and automate certain actions based on that data—and, with continuous monitoring and testing, and as new patterns and trends emerge, improve and optimize that machine wherever possible. In short: data analysts tackle and solve discrete questions about data, often on request, revealing insights that can be acted upon by other stakeholders, while data scientists build systems to automate and optimize the overall functioning of the business. Another main difference lies in the tools and skills required for each role. Data analysts are typically expected to be proficient in software like Excel and, in some cases, querying and programming languages like SQL, R, SAS, and Python. Analysts need to be comfortable using such tools and languages to carry out data mining, statistical analysis, database management and reporting. Data scientists, on the other hand, might be expected to be proficient in Hadoop, Java, Python, machine learning, and object-oriented programming, together with software development, data mining, and data analysis. Despite their differences, it’s important to recognize that data science and data analytics work together, and both make extremely valuable contributions to business.
3. What are the different types of data analysis?
Descriptive analytics
Descriptive analytics is a simple, surface-level type of analysis that looks at what has happened in the past. The two main techniques used in descriptive analytics are data aggregation and data mining—so, the data analyst first gathers the data and presents it in a summarized format (that’s the aggregation part) and then “mines” the data to discover patterns. The data is then presented in a way that can be easily understood by a wide audience (not just data experts). It’s important to note that descriptive analytics doesn’t try to explain the historical data or establish cause-and-effect relationships; at this stage, it’s simply a case of determining and describing the “what”. Descriptive analytics draws on descriptive statistics, which you can learn about here.Diagnostic analytics
While descriptive analytics looks at the “what”, diagnostic analytics explores the “why”. When running diagnostic analytics, data analysts will first seek to identify anomalies within the data—that is, anything that cannot be explained by the data in front of them. For example: If the data shows that there was a sudden drop in sales for the month of March, the data analyst will need to investigate the cause. To do this, they’ll embark on what’s known as the discovery phase, identifying any additional data sources that might tell them more about why such anomalies arose. Finally, the data analyst will try to uncover causal relationships—for example, looking at any events that may correlate or correspond with the decrease in sales. At this stage, data analysts may use probability theory, regression analysis, filtering, and time-series data analytics.Predictive analytics
Just as the name suggests, predictive analytics tries to predict what is likely to happen in the future. This is where data analysts start to come up with actionable, data-driven insights that the company can use to inform their next steps. Predictive analytics estimates the likelihood of a future outcome based on historical data and probability theory, and while it can never be completely accurate, it does eliminate much of the guesswork from key business decisions. Predictive analytics can be used to forecast all sorts of outcomes—from what products will be most popular at a certain time, to how much the company revenue is likely to increase or decrease in a given period. Ultimately, predictive analytics is used to increase the business’s chances of “hitting the mark” and taking the most appropriate action.Prescriptive analytics
Building on predictive analytics, prescriptive analytics advises on the actions and decisions that should be taken. In other words, prescriptive analytics shows you how you can take advantage of the outcomes that have been predicted. When conducting prescriptive analysis, data analysts will consider a range of possible scenarios and assess the different actions the company might take. Prescriptive analytics is one of the more complex types of analysis, and may involve working with algorithms, machine learning, and computational modeling procedures. However, the effective use of prescriptive analytics can have a huge impact on the company’s decision-making process and, ultimately, on the bottom line. The type of analysis you carry out will also depend on the kind of data you’re working with. If you’re not already familiar, it’s worth learning about the four levels of data measurement: Nominal, ordinal, interval, and ratio.4. What is data analytics used for?
The truth is, data is absolutely everywhere—which means that data analytics has almost infinite uses across all kinds of businesses and organizations. Broadly speaking, data analytics is used to make faster and more informed decisions, to reduce overall business costs, to develop more effective products and services, and to optimize processes and operations. In more specific terms, data analytics might be used for the following:
- To predict future sales and purchasing behaviors
- For security purposes—for example, to detect, predict, and prevent fraud within the insurance and banking industries
- To evaluate the effectiveness of marketing and advertising campaigns
- To optimize marketing efforts through more accurate targeting and personalization
- To identify and eliminate bottlenecks within a certain process
- To boost customer acquisition and retention
- To boost customer engagement on social media
- To develop risk management solutions
- To identify opportunities for innovation and create new revenue streams
- To increase supply chain efficiency
Data analytics case study: Healthcare
One area where data analytics is having a huge impact is the healthcare sector. Junbo Son, a researcher from the University of Delaware, has devised a system which helps asthma patients to better self-manage their condition using bluetooth-enabled inhalers and a special data analytics algorithm. So how does it work? First, the data is collected through a Bluetooth sensor which the user attaches to their asthma inhaler. Every time the patient uses their inhaler, the sensor transmits this usage data to their smartphone. This data is then sent to a server via a secure wireless network, where it goes through the specially devised Smart Asthma Management (SAM) algorithm. Over time, this unique algorithm helps to paint a picture of each individual patient, giving valuable insight into patient demographics, unique patient behaviours—such as when they tend to exercise and how this impacts their inhaler usage—as well as each patient’s sensitivity to environmental asthma triggers. This is especially useful when it comes to detecting dangerous increases in inhaler usage; the data-driven SAM system can identify such increases much more quickly than the patient would be able to. What’s more, the SAM system has been found to outperform traditional models, with a false alarm rate that is 10-20% lower than that of current models, together with a 40-50% lower misdetection rate. This case study highlights what a difference data analytics can make when it comes to providing effective, personalized healthcare. By collecting and analyzing the right data, healthcare professionals are able to offer support that is tailored to both the individual needs of each patient and the unique characteristics of different health conditions—an approach that could be life-changing and potentially life-saving. You can learn more about this case study in the following journal article: A Data Analytics Framework for Smart Asthma Management Based on Remote Health Information Systems with Bluetooth-Enabled Personal Inhalers.
Data analytics case study: Netflix
Another real-world example of data analytics in action is one you’re probably already familiar with: the personalized viewing recommendations provided by Netflix. So how does Netflix make these recommendations, and what impact does this feature have on the success of the business? As you might have guessed, it all starts with data collection. Netflix collects all kinds of data from its 163 million global subscribers—including what users watch and when, what device they use, whether they pause a show and resume it, how they rate certain content, and exactly what they search for when looking for something new to watch. With the help of data analytics, Netflix are then able to connect all of these individual data points to create a detailed viewing profile for each user. Based on key trends and patterns within each user’s viewing behavior, the recommendation algorithm makes personalized (and pretty spot-on) suggestions as to what the user might like to watch next. This kind of personalized service has a major impact on the user experience; according to Netflix, over 75% of viewer activity is based on personalized recommendations. This powerful use of data analytics also contributes significantly to the success of the business; if you look at their revenue and usage statistics, you’ll see that Netflix consistently dominates the global streaming market—and that they’re growing year upon year. As you can see from these two case studies alone, data analytics can be extremely powerful. For more real-world case studies, check out these five examples of how brands are using data analytics—including how Coca Cola uses data analytics to drive customer retention, and how PepsiCo uses their huge volumes of data to ensure efficient supply chain management.